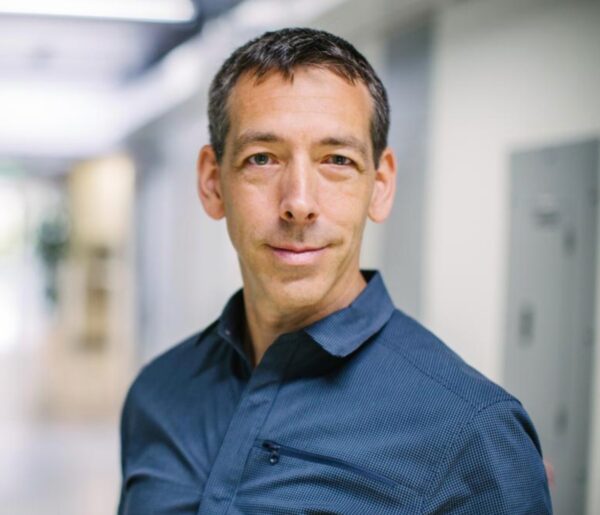
Euan Ashley is an associate dean of the School of Medicine, the Roger And Joelle Burnell Professor of Genomics and Precision Health, and a professor of medicine, of genetics, and of biomedical data science. Ashley’s research group is focused on the science of precision medicine. In 2010, he led the team that carried out the first clinical interpretation of a human genome.
New methods such as genomic sequencing and mass spectrometry have prompted dramatic increases in the amount of molecular data available to scientists and health care professionals seeking more refined diagnoses and increased therapeutic precision. Although the largest advances have been made in genetic sequencing of DNA and RNA, medical applications of high-dimensional measurement of proteins and metabolites are increasing.
Analytic tools have been improved in parallel to match the volume, velocity, and variety of these molecular “big data.” The emergence of #MachineLearning has proved especially valuable. In these approaches, computer systems use large amounts of data to build predictive statistical models that are iteratively improved by incorporating new data. Deep learning, a powerful subset of machine learning that includes the use of deep neural networks, has had high-profile applications in image object recognition, voice recognition, autonomous driving, and virtual assistance. These approaches are now being applied in medicine to yield clinically directive medical information. In this review article, we briefly describe the methods used to generate high-dimensional molecular data and then focus on the key role that machine learning plays in the clinical application of such data.
Machine-learning methods for analyzing genomic, transcriptomic, epigenomic, proteomic, and metabolomic data sets have yielded clinically directive information, mostly for rare genetic diseases.